Enhancing Ethical AI Use: Solutions, Examples, and Innovations
- Oct 15, 2024
- 3 min read
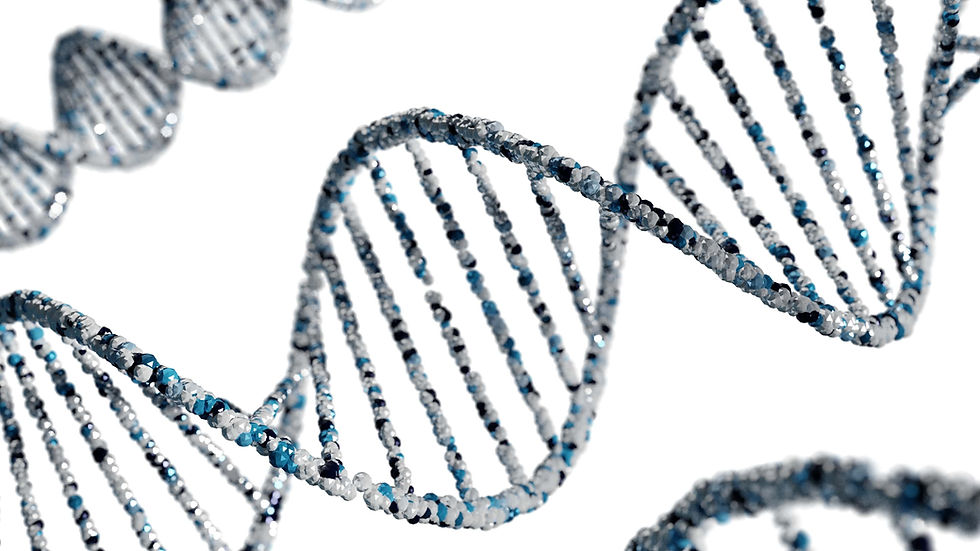
AI systems have transformed industries, from healthcare to finance. However, issues like bias, lack of transparency, and overreliance create challenges that demand ethical solutions. This article explores real-world examples of these problems and proposes innovative solutions that build on existing frameworks.
---
Real-World Examples of Ethical Challenges
1. **Healthcare**: In clinical settings, AI tools such as diagnostic algorithms have shown potential to enhance outcomes. However, overreliance on AI has caused problems. For example, studies show that clinicians sometimes defer to AI recommendations even when those recommendations are incorrect, compromising patient outcomes. This highlights the need for better trust calibration in AI-assisted decision-making to ensure medical practitioners can discern when to rely on human expertise over machine predictions.
2. **Finance**: AI-driven lending models have also faced scrutiny for perpetuating biases. Certain algorithms have been found to deny loans disproportionately to minority applicants, even when they are financially qualified. These biases result from the use of historical data that reflects systemic discrimination, leading to unjust outcomes.
3. **Criminal Justice**: Predictive policing tools have been criticized for reinforcing racial biases. In Chicago, the use of such tools increased the targeting of specific communities based on biased historical arrest data, raising ethical questions about fairness and transparency.
---
Innovative Ethical Solutions to Existing Issues
1. **Cognitive Forcing Functions in AI-Assisted Decisions**
Cognitive forcing functions are a promising intervention to prevent overreliance on AI. These functions disrupt fast, intuitive decision-making processes, forcing users to engage analytically with the task at hand. For example, healthcare professionals can use diagnostic time-outs to cross-check AI recommendations, a technique that has been proven effective in clinical settings. Similarly, adaptive checklists and slower decision-making protocols can be employed in AI-assisted lending to improve judgment and fairness in financial decisions.
2. **Bias Auditing and Transparent AI Development**
Regular bias audits of AI systems can help mitigate unfair outcomes. This can involve third-party audits to ensure accountability, especially in high-stakes fields such as finance and criminal justice. Transparency frameworks that provide clear explanations for AI decisions, such as model interpretability tools, enable users to understand the logic behind outputs and detect potential biases.
3. **Hybrid AI Systems with Calibrated Trust Mechanisms**
Building hybrid systems where humans and AI work collaboratively requires trust calibration. Techniques such as confidence scoring and explainable AI (XAI) can improve decision quality. For example, XAI in medical AI systems can provide confidence levels alongside predictions, encouraging clinicians to question low-confidence recommendations while trusting high-confidence ones appropriately.
4. **Algorithmic Fairness Regulations and Monitoring Platforms**
Governments and industry stakeholders need to implement regulations that enforce fairness. Algorithmic impact assessments and continuous monitoring platforms can track and measure the fairness of deployed AI systems over time. These assessments should involve diverse stakeholder input to ensure they reflect the concerns of various communities affected by AI.
---
Proposed Innovations
1. **Ethical AI Certification Programs**: Developing AI-specific certification programs similar to quality certifications in other industries can ensure companies follow best ethical practices. This can also serve as a market differentiator, encouraging ethical innovation.
2. **Personalized AI Trust Calibration**: Implementing personalized trust systems, where users receive training on how to interact with specific AI models, can enhance trust calibration. For example, a financial advisor using AI-powered investment tools could receive tailored feedback to align their decisions with the AI’s strengths while mitigating weaknesses.
3. **Ethical Digital Twins for Testing and Development**: Before deploying new AI tools, organizations could use digital twin environments—virtual replicas of the real world—to simulate the ethical impact of AI in practice. This proactive testing approach helps address potential risks and biases before they affect real users.
4. **Incentive Structures for Ethical AI Development**: Introducing incentive structures that reward organizations for meeting ethical benchmarks—such as lower bias rates and higher transparency—can motivate companies to prioritize ethics alongside performance.
---
These solutions address the complex interplay between AI performance, human decision-making, and ethics, aiming for more balanced and fair outcomes across industries. Implementing these strategies can help overcome current challenges and build a foundation for more responsible AI use in the future.
References:
- Research on cognitive forcing functions and human-AI collaboration shows how structured thinking can mitigate overreliance on AI (ar5iv.org).
- Discussions on bias in AI, transparency, and regulation highlight the importance of algorithmic fairness in industries such as finance and law (ar5iv.org).
- Case studies on predictive policing and AI lending models reveal the risks of uncorrected biases and the need for robust audit processes.
Comments